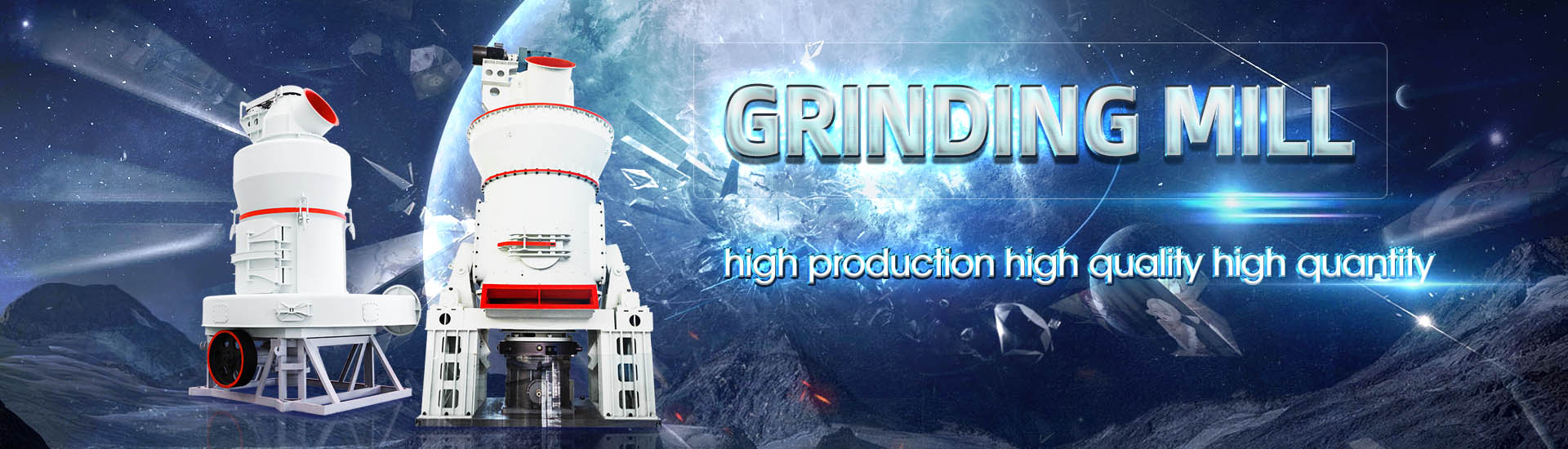
Dynamic powder selection machine

Dynamic coal milling powderselecting machine Google Patents
Dynamic coal milling powderselecting machine, it is characterized in that: this device contour structures is a upper cone, middle cylindrical shell, lower cone is formed byBy retrieval, China's utility model patent 4 discloses a kind of dynamic coal milling powderselecting machine, the hypomere of this device is inside and outside double layerDynamic coal milling powderselecting machine Google PatentsThe utility model provides a dynamic powder concentrator, has solved the current powder concentrator when using the air current the water conservancy diversion direction relatively fixed,CNU Dynamic powder selecting machine Google There are fine powder airflow outlet in the upper cone, coal powder airflow inlet in the lower cone, and inner conic barrel inside the lower cone and connected to the lower coarse powder outlet The present invention has the advantages of raised coal powder fineness, raised coal powder yield, and lowered power consumptionDynamic coal milling powderselecting machine Semantic Scholar
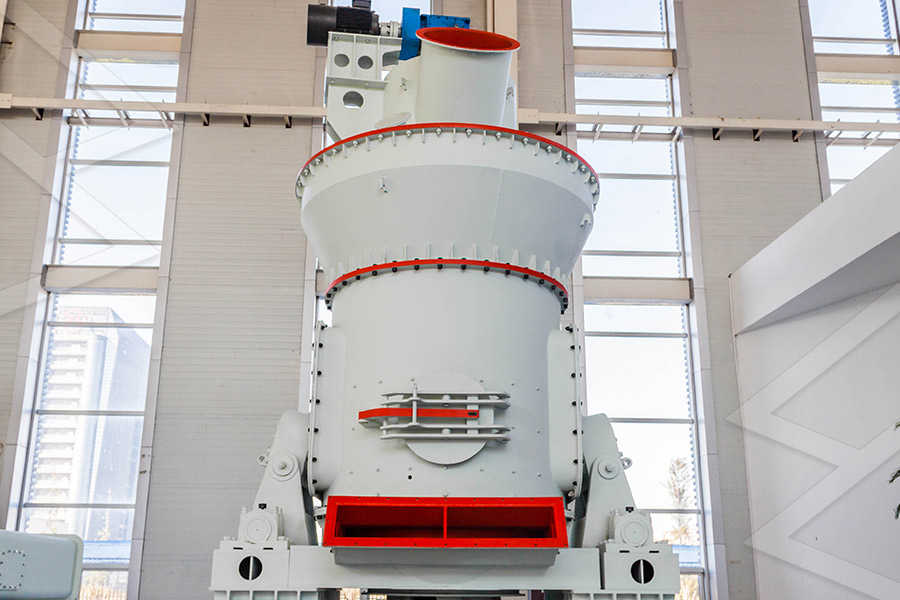
Advanced Powder Technology
2023年6月1日 In this paper, a numerical calculation method for internal particle–fluid coupling system of large vertical mills based on CFDDPM theory and a multiobjective optimization method for operating parameters of large vertical mills based on kriging are proposed, and the response value of sample points is calculated by multiphysics coupling 2016年10月18日 Adopting dynamic powder flow measurement with the FT4 Powder Rheometer has proved highly efficient, compared to alternative powder testing options, and provides highly relevant data for the selection of Using Dynamic Powder Testing to Optimize Packaging 2020年1月1日 A dynamic powder splitting system (DPSS) was developed to overcome a slow powder flow rate (PFR) response in conventional powder delivery systems used in the Directed Energy Deposition (DED) additive manufacturing processDevelopment and evaluation of a dynamic powder splitting 2015年7月8日 This article describes new collaborative research from Freeman Technology and Gericke to establish predictive correlations for the volumetric flow rate delivered by screw feeders, using dynamic powder testing that can be Predicting Feeder Performance from Powder Flow
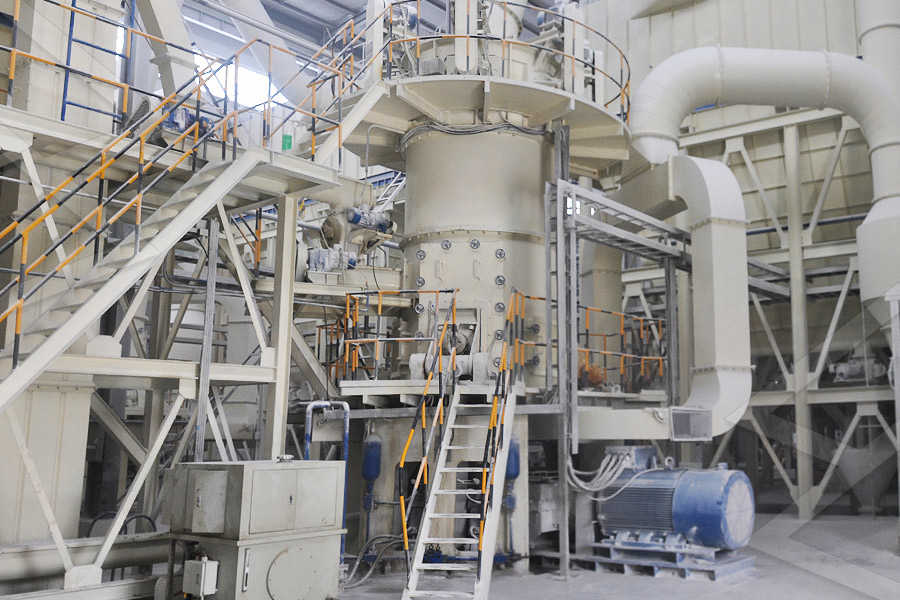
Powder characterization for effective powder processing
2020年4月6日 Investigating the correlation between powder properties and performance in a specific unit operation is helpful in illustrating the application of multifaceted powder characterization and the value of dynamic powder testing2023年8月2日 The selection of powder packaging machines is vast, including specialized machines like the small powder filling machine for low volume needs, the automatic powder packaging machine for high production requirements, Selecting Guide for Powder Packing Machine Great 2023年7月26日 The study results indicate that machine learning techniques can provide a more accurate and efficient approach to wind power plant site selection compared to traditional methodsWind Power Plant Site Selection using Integrated 2022年5月25日 Nowadays, the proportional–integral–derivative (PID) controller dominates industrial process control Because of the compromise between parameters, its tuning is still a challenge for practitioners A reference model Research on the Desired Dynamic Selection of a
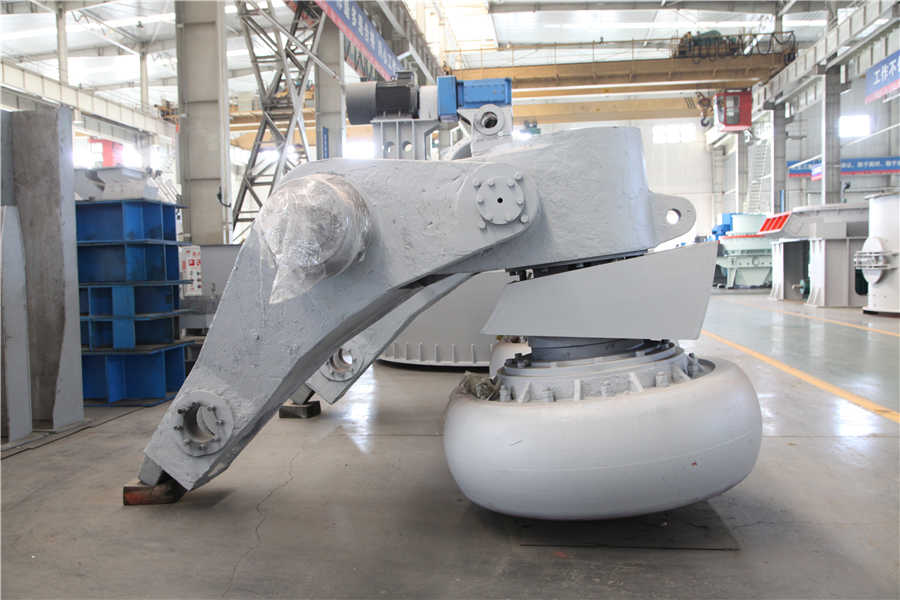
Dynamic Power Management Using Machine Learning
2006年11月1日 Another example of a model selection mechanism is [22], where Dhiman et al implements a dynamic device management controller that employs a machine learning algorithm, which is trained to select 2018年2月14日 Powder packing structure is a critical parameter of powder bedbased additive manufacturing (AM) Experimental characterization of powder is typically limited to measuring bulk properties, whereas many numerical models of AM powder packing are based on geometrical consideration without accounting for particletoparticle interactions In the present paper, the Dynamic simulation of powder packing structure for powder 2024年2月15日 The dynamic flowability was characterized by OMR, and its linear dependency on the PSD was illustrated in Fig 13 A positive linear relationship with dynamic powder flowability was observed when the particle diameter was less than 0 1 µ mDecoding powder flowability: Machine learning pioneers the 2024年11月11日 Laser powder bed fusion (LPBF) additive manufacturing at German 3D printer OEM EOS Photo via EOS The AMS factor Freeman Technology’s FT4 Powder Rheometer is a universal powder flow tester for New research proposes AM Suitability factor for metal powder selection
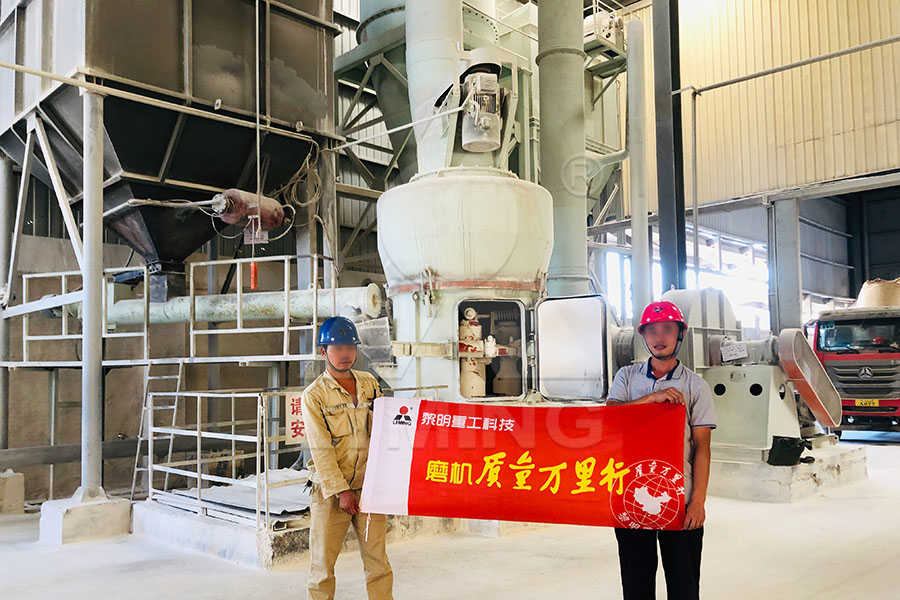
Predicting Feeder Performance from Powder Flow Measurements
2015年7月8日 Introducing Dynamic Powder Testing The FT4 Powder Rheometer enables the measurement of bulk, shear and, uniquely, dynamic powder properties As a result, it allows a powerful, multifaceted approach to powder testing that has proven extremely valuable for identifying powder properties that correlate robustly with aspects of process performance2022年12月1日 Dynamic model selection is an active research field with applications in many areas such as construction (Azad et al, 2022), photonic computing (Kanno et al, 2020), cloud network analytics (Karn et al, 2019) and renewable energies (Kosana et al, 2022), among othersThe most commonly used approach for implementing dynamic model selection is A twostep machine learning approach for dynamic model selection2022年12月1日 PDF Generally, dynamic model selection is implemented using algorithms that need a feedback from the system’s output; but, in many realworld Find, read and cite all the research you need A twostep machine learning approach for dynamic model selection2023年10月27日 At the same time, the powder selection machine should have good adaptability and be suitable for different types and characteristics of cellulose materials Only in this way can powder separator suitable for different cellulose materials How to choose a powder separator suitable for different cellulose
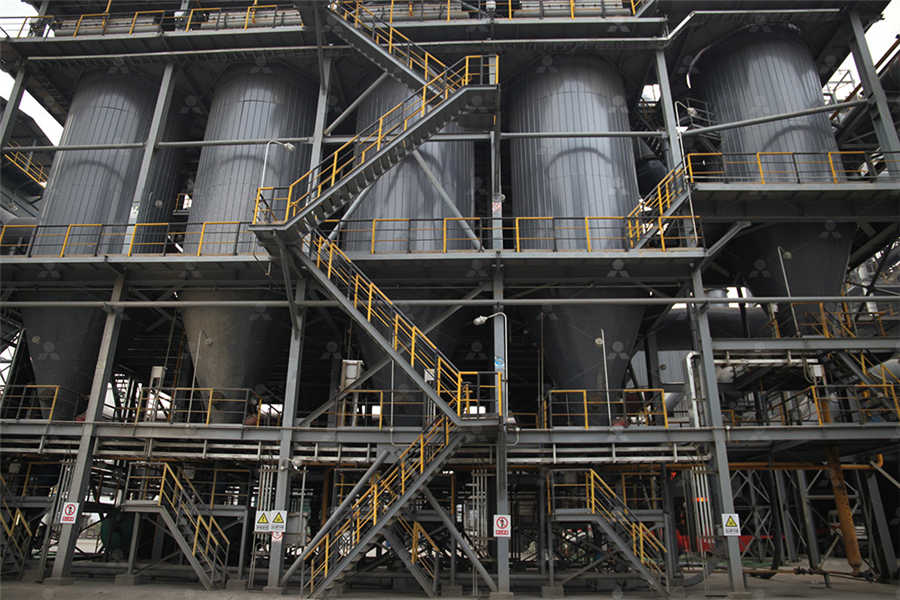
SmartDPM: Machine LearningBased Dynamic Power
and nearly 15% higher power savings on average compared to the existing machine learning based power management schemes for a microprocessor with up to 32cores Keywords: MultiCore Microprocessor, Power Management, Machine Learning, Dynamic Voltage Frequency Scaling, Prediction, Control Theory, Online Learning, Scalability, Energy Efficiency 12021年4月27日 Dynamic ensemble selection is an ensemble learning technique that automatically selects a subset of ensemble members justintime when making a prediction The technique involves fitting multiple machine learning Dynamic Ensemble Selection (DES) for Nonorthogonal multiple access (NOMA) is considered a potential technique in fifthgeneration (5G) Nevertheless, it is relatively complex when applying NOMA to a massive access scenario Thus, in this paper, a hybrid NOMA/OMA scheme is considered for uplink wireless transmission systems where multiple cognitive users (CUs) can simultaneously transmit their data to a Hybrid NOMA/OMABased Dynamic Power Allocation Scheme Published by Maney Publishing (c) IOM Communications Ltd An overview of dynamic compaction in powder metallurgy G Sethi*1, N S Myers2 and Randall M German3 This paper is a critical review of An overview of dynamic compaction in powder metallurgy
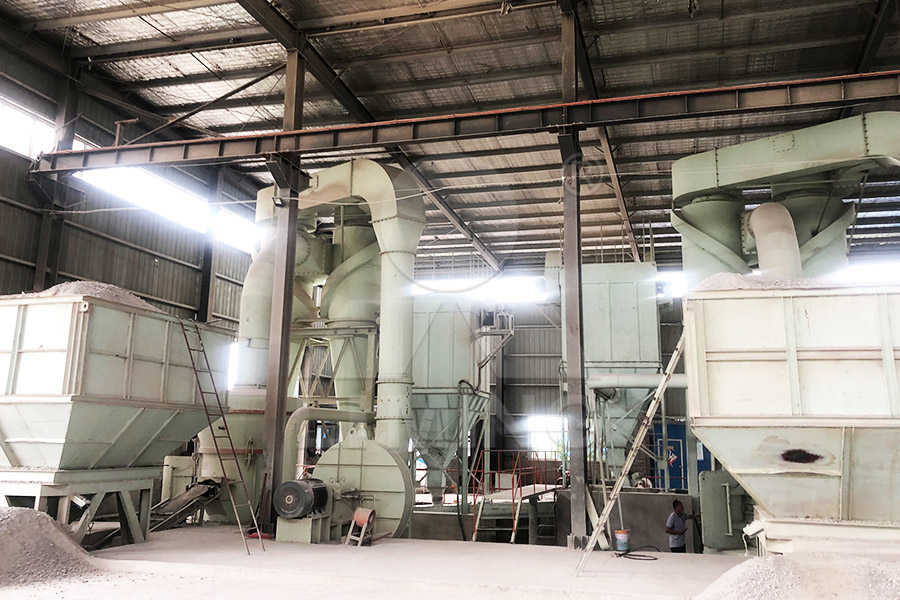
SmartDPM: Machine LearningBased Dynamic Power
2018年12月1日 Request PDF SmartDPM: Machine LearningBased Dynamic Power Management for MultiCore Microprocessors To address the power management challenge in multicore microprocessors, we present a Stock recommendation is vital to investment companies and investors However, no single stock selection strategy will always win while analysts may not have enough time to check all SP 500 stocks (the Standard Poor’s 500) In this paper, we propose a practical scheme that recommends stocks from FinML: A Practical Machine Learning Framework for Dynamic Stock Selection2023年10月7日 Metal Powder Bed Fusion (MPBF) technique is one of the popular branches of Additive Manufacturing (AM) One of the biggest challenges in MPBF is understanding relationship between processing parameters and produced part’s mechanical properties In this review paper, recent MPBF and Machine Learning (ML) studies are comparatively A Critical Review of Machine Learning Methods Used in Metal Powder 2021年5月28日 A quick post today about how to use RANKX based on slicer selection in Power BI A RANKX return the ranking of a number for each row in a table The following DAX rank the Sales Amount for each Product and works fine without a slicer = RANKX(ALL(Products), SUMX(RELATEDTABLE(InternetSales), [SalesAmount])) The solution below will show how to DYNAMIC RANKX BY SLICER SELECTION IN POWER BI
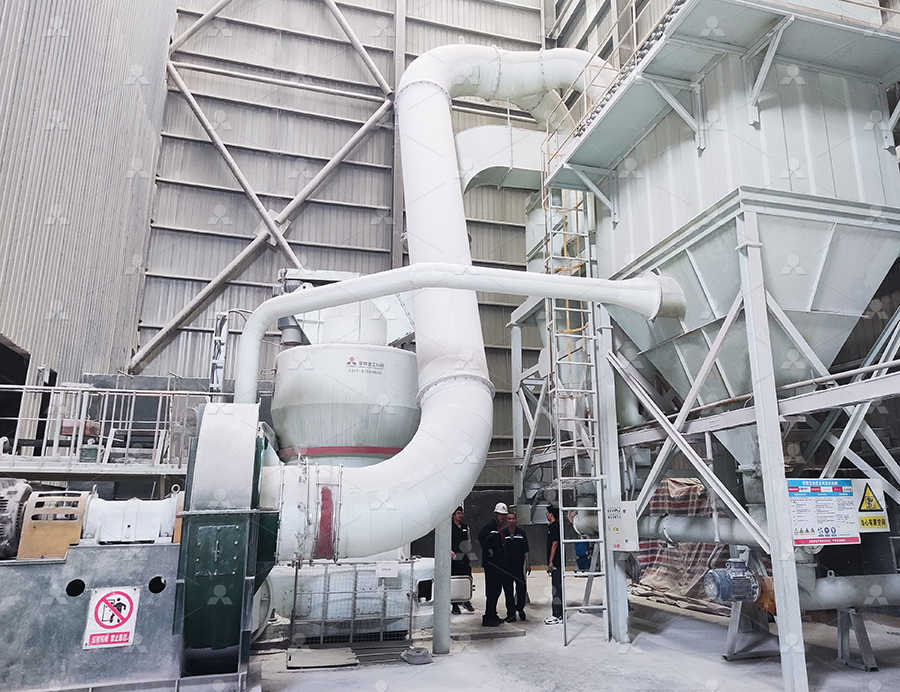
Enhancing Machine Learning Prediction in Cybersecurity Using Dynamic
Machine learning algorithms are becoming very efficient in intrusion detection systems with their real time response and adaptive learning process A robust machine learning model can be deployed for anomaly detection by using a comprehensive dataset with multiple attack types Nowadays datasets contain many attributes Such high dimensionality of datasets poses a Wind Power Plant Site Selection using Integrated Machine Learning and MultipleCriteria Decision Making Technique Patrick D Cerna1, Ryan S Evangelista2, Cromwell M Castillo3, Jehana A Muallam Darkis4, Mark Anthony C Velasco5, John P Legaspi6, Aldaruhz T Darkis7, Ma Magdalena Gatdula8 1Negros State College of Science and Technology, PhilippinesWind Power Plant Site Selection using Integrated Machine 2021年4月7日 Powder Mixer Selection Guide How to choose a right pharmaceutical mixer? Wednesday, April 7, 2021 (Dynamic Angle of Repose ≤ 45°), Powder Mixing Machine Tablet Coating Machine Blister Packaging Powder Mixer Selection Guide IPharmachineDownload Table Dynamic compaction machine parameters: different machines have different energy carriers and controlling parameters 32–34 from publication: An overview of dynamic compaction in Dynamic compaction machine parameters: different
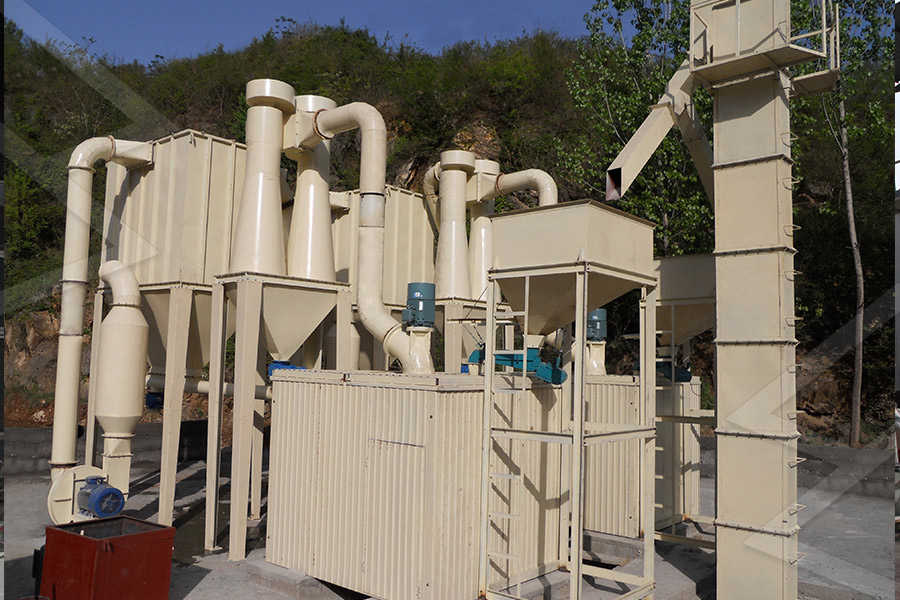
An overview of dynamic compaction in powder metallurgy
2008年7月1日 Powder related factors include powder characteristics and the processing parameters including the machine operating parameters Attention has been given to iron, aluminium and copper powdersDynamic Data Selection for Neural Machine TranslationAuthors: Marlies van der Wees, Arianna Bisazza, Christof MonzOrganizations: Informatics Institute University of Amsterdam, LIACS Leiden UniversitDynamic Data Selection for NMT 知乎2020年10月20日 Fast and accurate online dynamic security analysis (DSA) is the key enabler for secure operation of modern power systems Realtime assessment of the current power system operating state and increased awareness about plausible future insecurity can enable necessary operational and control measures to ensure secure operation This paper proposes Realtime dynamic security analysis of power systems using 2017年12月28日 In the present paper, a new powerful tool is developed to find the dynamic stability margin of multimachine power system The dynamic stability margin is evaluated by finding the eigenvalues of the power system ‘A’ matrix under different loading conditionsThe loading at which the system exhibits imaginary pair of eigenvalues is the dynamic stability Dynamic Stability Margin Evaluation of Multimachine Power
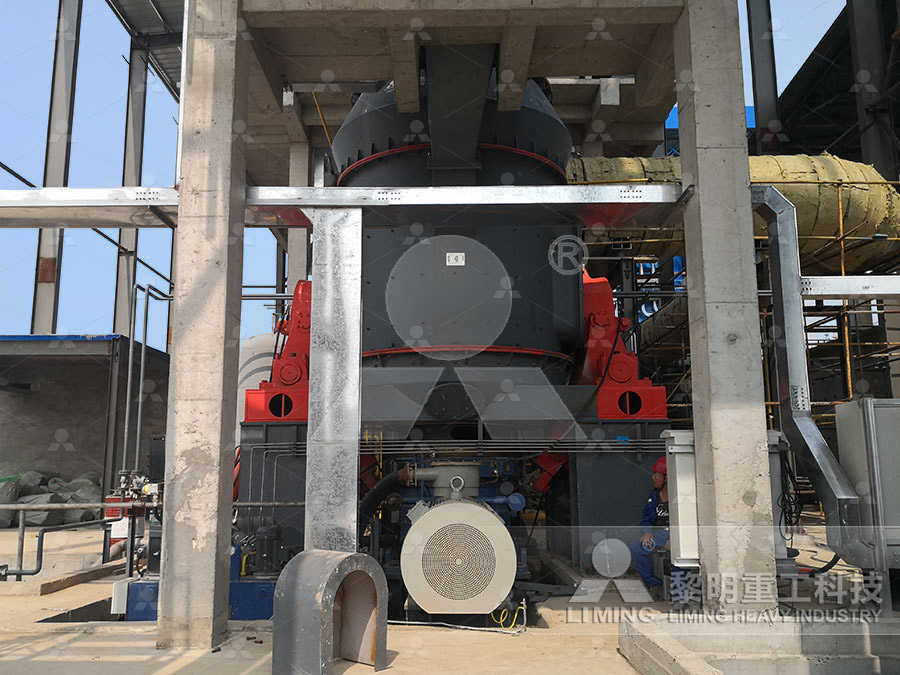
Virtual machine selection and placement for dynamic consolidation
Dynamic consolidation of virtual machines (VMs) in a data center is an effective way to reduce the energy consumption and improve physical resource utilization Determining which VMs should be migrated from an overloaded host directly influences the VM migration time and increases energy consumption for the whole data center, and can cause the service level of agreement (SLA), 2015年2月11日 Dynamic consolidation of virtual machines (VMs) in a data center is an effective way to reduce the energy consumption and improve physical resource utilization Determining which VMs should be migrated from an overloaded host directly influences the VM migration time and increases energy consumption for the whole data center, and can cause the service level Virtual machine selection and placement for dynamic Dynamic Test Case Selection using Machine Learning Dynamisk Selektion av Testfall med Maskininlärning Jesper Larsson Niklas Höstklint Examensarbete inom Datateknik, Grundnivå, 15 hp Handledare på KTH: Jayanth Raghothama Examinator: Ibrahim Dynamic Test Case Selection using Machine Learning2023年8月2日 The selection of powder packaging machines is vast, including specialized machines like the small powder filling machine for low volume needs, the automatic powder packaging machine for high production requirements, Selecting Guide for Powder Packing Machine Great
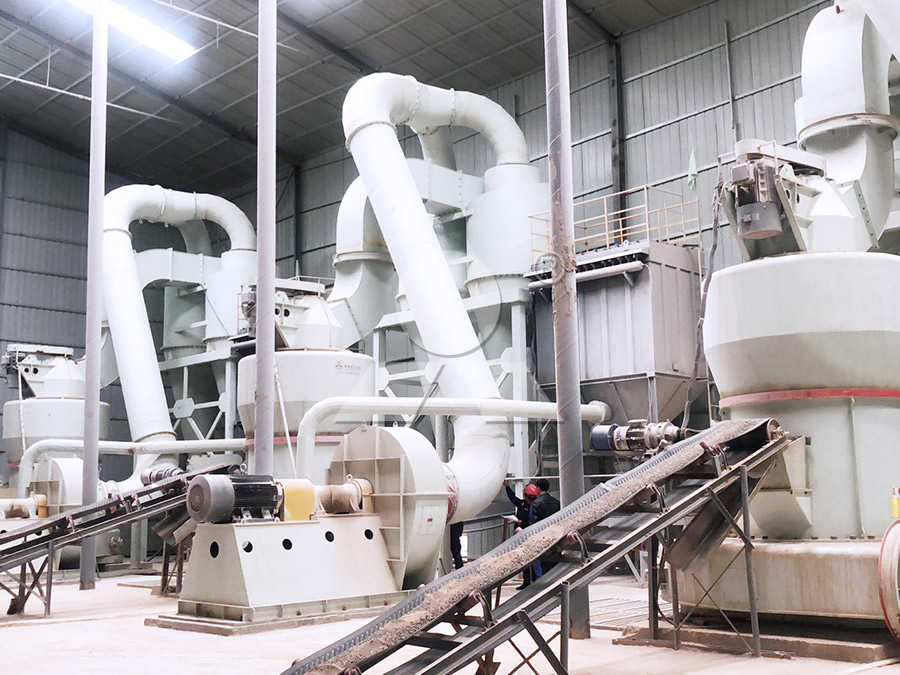
Wind Power Plant Site Selection using Integrated
2023年7月26日 The study results indicate that machine learning techniques can provide a more accurate and efficient approach to wind power plant site selection compared to traditional methods2022年5月25日 Nowadays, the proportional–integral–derivative (PID) controller dominates industrial process control Because of the compromise between parameters, its tuning is still a challenge for practitioners A reference model Research on the Desired Dynamic Selection of a 2006年11月1日 Another example of a model selection mechanism is [22], where Dhiman et al implements a dynamic device management controller that employs a machine learning algorithm, which is trained to select Dynamic Power Management Using Machine Learning2018年2月14日 Powder packing structure is a critical parameter of powder bedbased additive manufacturing (AM) Experimental characterization of powder is typically limited to measuring bulk properties, whereas many numerical models of AM powder packing are based on geometrical consideration without accounting for particletoparticle interactions In the present paper, the Dynamic simulation of powder packing structure for powder
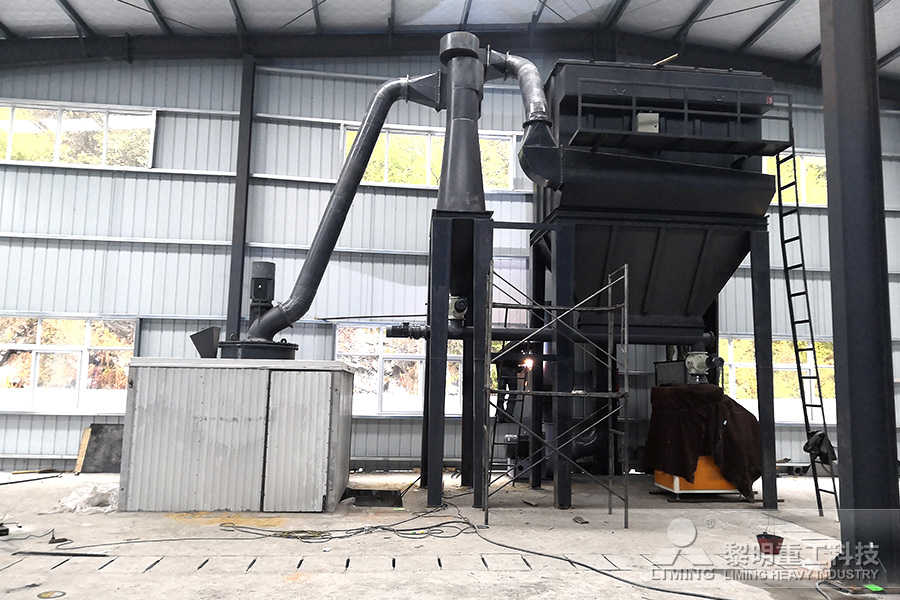
Decoding powder flowability: Machine learning pioneers the
2024年2月15日 The dynamic flowability was characterized by OMR, and its linear dependency on the PSD was illustrated in Fig 13 A positive linear relationship with dynamic powder flowability was observed when the particle diameter was less than 0 1 µ m2024年11月11日 Laser powder bed fusion (LPBF) additive manufacturing at German 3D printer OEM EOS Photo via EOS The AMS factor Freeman Technology’s FT4 Powder Rheometer is a universal powder flow tester for New research proposes AM Suitability factor for metal powder selection2015年7月8日 Introducing Dynamic Powder Testing The FT4 Powder Rheometer enables the measurement of bulk, shear and, uniquely, dynamic powder properties As a result, it allows a powerful, multifaceted approach to powder testing that has proven extremely valuable for identifying powder properties that correlate robustly with aspects of process performancePredicting Feeder Performance from Powder Flow Measurements2022年12月1日 Dynamic model selection is an active research field with applications in many areas such as construction (Azad et al, 2022), photonic computing (Kanno et al, 2020), cloud network analytics (Karn et al, 2019) and renewable energies (Kosana et al, 2022), among othersThe most commonly used approach for implementing dynamic model selection is A twostep machine learning approach for dynamic model selection
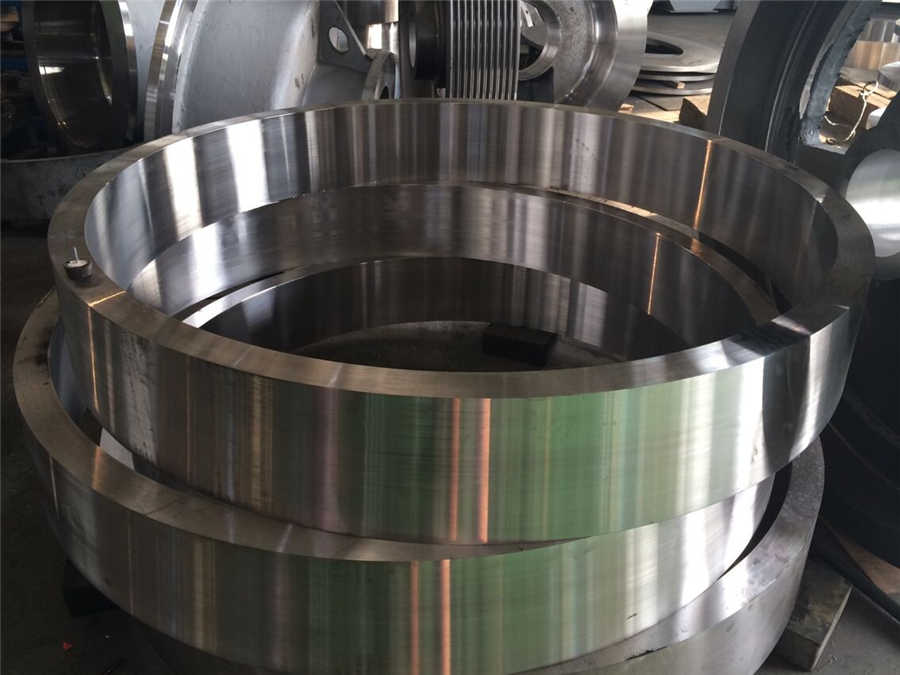
A twostep machine learning approach for dynamic model selection
2022年12月1日 PDF Generally, dynamic model selection is implemented using algorithms that need a feedback from the system’s output; but, in many realworld Find, read and cite all the research you need